AI-Driven Data Processing
Many of the devices produced in the center make use of new sensing modalities (e.g., detecting glucose from sweat) and will be integrated in possibly innovative form factors (e.g., integrated in textiles). The data coming from these systems can demonstrate unexpected artifacts (e.g., based on motion) that need to be understood in order to extract reliable biomarkers. Standard signal processing pipelines often rely on assumptions about the structure of the signals and the nuisances. Furthermore, extraction of relevant biomarkers from physiological signals may require the identification of complex non-linear relationships.
Machine learning models are been developed that aim to capture these complex relationships and determine the context under which physiological signals are reliable enough to extract the desired biomarkers. The context may depend on environmental factors, and the states of the user and the devices. The reliability of the signals can be captured using data-driven signal quality indexes which are modeled using deep learning models that incorporate uncertainty quantification. This information can be used to inform the user when data is not reliable or to set parameters in the device.
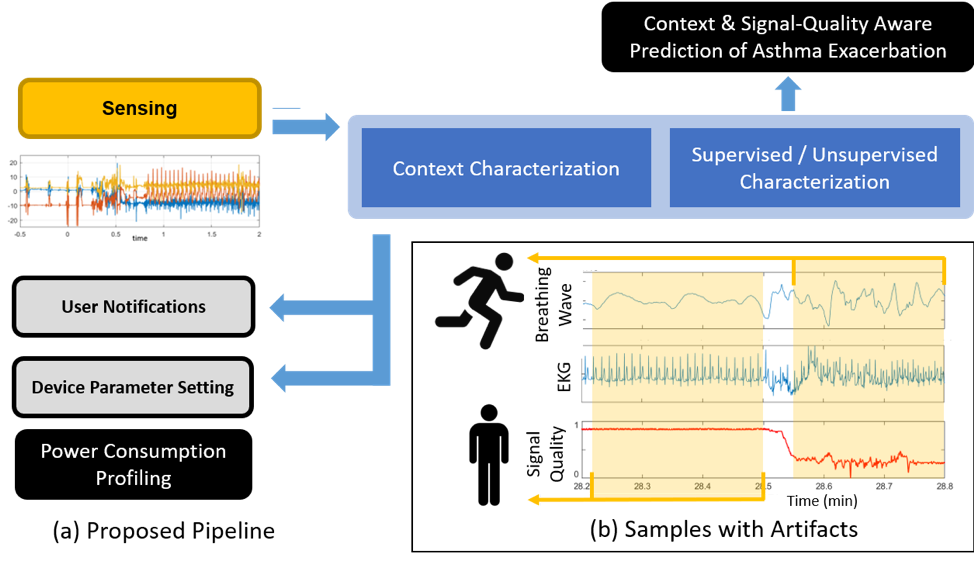